Mina Valizadeh defends her PhD thesis
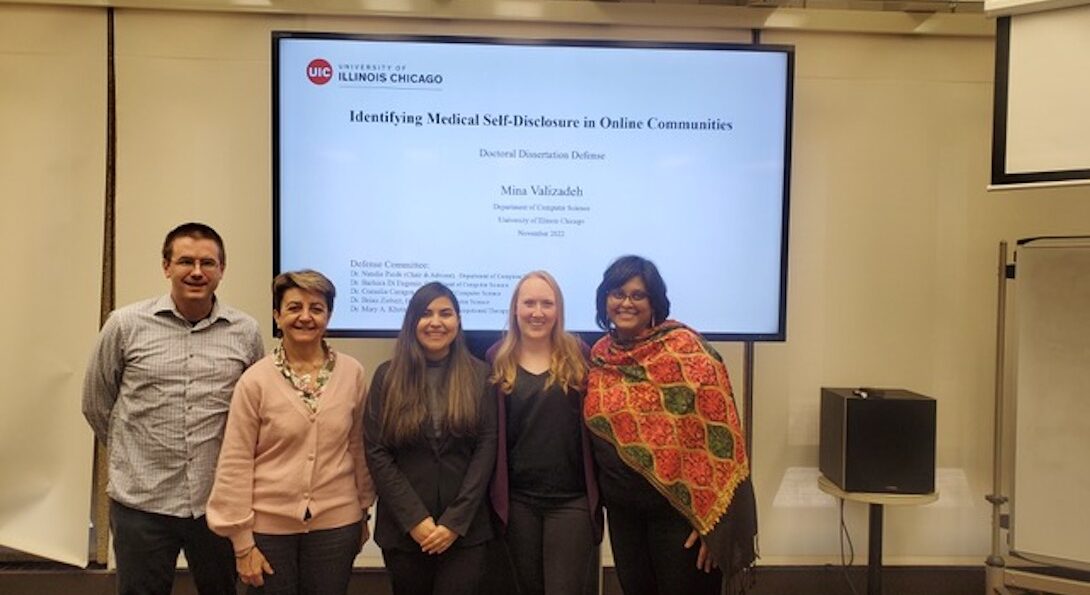
Congratulations to Mina Valizadeh for successfully defending her PhD thesis on November 21, 2022! The title of her thesis is "Identifying Medical Self-Disclosure in Online Communities".
Mina's committee members included: Natalie Parde (advisor; CS, UIC), Barbara Di Eugenio (CS, UIC), Cornelia Caragea (CS, UIC), Brian Ziebart (CS, UIC), and Mary Khetani (Department of Occupational Therapy, UIC).
Abstract:
Medical self-disclosure is the communicative act of sharing personal information regarding medical symptoms, medications, diagnoses, or related content. Paradoxically, it may occur more frequently in online, potentially anonymous settings than in conversation with a trained physician. Disclosing health information may lead directly or indirectly to a variety of benefits, including earlier detection and treatment of latent or otherwise unaddressed medical issues; however, before benefits can be reaped, these disclosures must be recognized.
Research towards detecting and analyzing online medical self-disclosure to date has been limited. In this dissertation, we address this shortcoming by establishing the novel task of automatically detecting medical self-disclosure. We introduce a large, publicly available dataset of health-related posts collected during a two-stage annotation process from online social platforms, annotated with graded (No Self-Disclosure, Possible Self-Disclosure, and Clear Self-Disclosure) labels pertaining to medical self-disclosure specifically. We manually refine and clinically validate the dataset, ensuring high quality and validity. Our initial experiments aimed at broad model comparison and task validation achieve a classification accuracy of 76.77%, establishing a strong preliminary performance benchmark. Following our establishment of dataset and task validity, we conduct comprehensive follow-up work to study and systematically analyze model performance and behaviors for medical self-disclosure detection.
First, we investigate the merits of pretraining task domain and text style by comparing Transformer-based models pretrained on a variety of general, medical, and social media sources and fine-tuned for this task. We find that a fine-tuned BERTweet model outperforms our earlier state-of-the-art by a substantial relative F-1 score increase of 16.73%, suggesting that stylistic attributes carry more importance than purely domain-specific expertise when recognizing medical self-disclosure. We also assess the relationship between performance and dataset size under varying conditions. We measure the relationship between manually-created dataset size and performance by training on gradually increasing samples of the final version of our dataset, and we measure the capacity of synthetic data to extend performance beyond that observed with manual data alone by empirically comparing a suite of data augmentation techniques. Our study of data augmentation for medical self-disclosure detection reveals many challenges associated with generating useful synthetic data to support performance for this task, and we provide an in-depth analysis of identified trends.
Next, we investigate the extent to which transfer learning from conceptually relevant source tasks (i.e., emotion recognition or figurative language detection) or multi-task learning leveraging these tasks as auxiliary tasks can positively influence medical self-disclosure detection performance. We find that our multi-task learning model trained using EmoNet (an emotion recognition task) as an auxiliary task resulted in a small but distinct performance improvement. This model is the new state-of-the-art for our challenging multinomial medical self-disclosure detection task (accuracy=88.13% and F-1 score=0.8589).
As a proof of concept, we also design the first regression model for scoring medical self-disclosure along a spectrum from No Self-Disclosure to Clear Self-Disclosure. Results from a performance evaluation demonstrate convincing performance for our model (RMSE=0.9034 and MAE=0.6339). We encourage researchers to further explore this task as a challenging next step for future work. Finally, we conclude this dissertation by discussing feasible real-world applications of our implemented models and revealing exciting directions for follow-up work by others.